New CAS Research Paper on Deep Learning Forecasts for Individual Claims
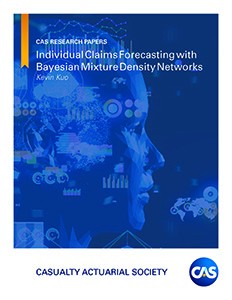
The Casualty Actuarial Society recently published a new piece of research with potential immediate applications in individual claims forecasting. Part of the new CAS Research Paper series, “Individual Claims Forecasting with Bayesian Mixture Density Networks” is written by Kevin Kuo and introduces an individual claims forecasting framework utilizing Bayesian mixture density networks; the paper outlines a modeling framework that uses a publicly available data simulation tool.
The methods shown have immediate utility in claims departments, where case adjusters and actuaries may compare scenarios and estimates for the progression of individual claims. The research also demonstrates a practical application of deep learning in an actuarial/insurance context.
The paper is part of the CAS Research Paper series, which are funded, peer-reviewed, in-depth works focusing on important topics within property-casualty actuarial practice. These papers give CAS members access to relevant information and resources applicable to their work, which can help advance their careers. Funding for CAS Research Papers comes from CAS member dues, individual grants and other sources. For additional information, visit the CAS Research Papers page