About the CAS Monographs Series
CAS monographs are authoritative, peer-reviewed, in-depth works focusing on important topics within property and casualty actuarial practice. The inaugural monograph, Stochastic Loss Reserving Using Bayesian MCMC Models, was published in January 2015.
The CAS Monograph Series initiative fulfills the goal of creating an important addition to the existing body of CAS literature, with each monograph enabling the comprehensive treatment of a single subject.
The monographs represent just one way that the CAS provides its members with access to relevant information, research and resources that they can apply directly on the job to advance in their careers.
Published Monographs
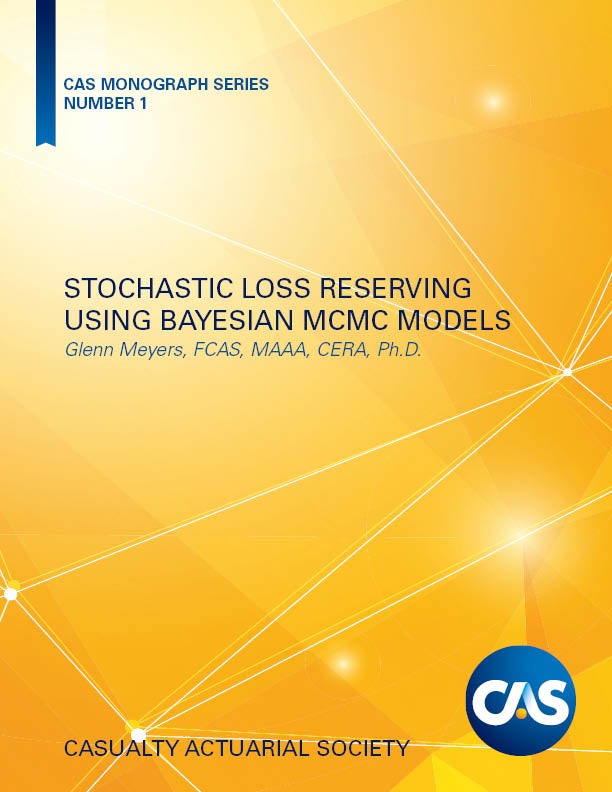
CAS Monograph No. 1 –Stochastic Loss Reserving Using Bayesian MCMC Models
by Glenn Meyers, FCAS, MAAA, CERA
In this monograph, Glenn Meyers introduces a novel way of testing the predictive power of two loss reserving methodologies. Using a database created by the CAS that consists of hundreds of loss development triangles with outcomes, the volume begins by first testing the performance of the Mack model on incurred data and the Bootstrap Overdispersed Poisson model on paid data. As the emergence of Bayesian MCMC models has provided actuaries with an unprecedented flexibility in stochastic model development, the monograph then identifies some Bayesian MCMC (Markov Chain Monte Carlo) models that improve the performance over the above models. PLEASE NOTE that there is now a revised edition of this monograph (Monograph #8).
The monograph has been adopted on the syllabus for Exam 7.
In this monograph, Glenn Meyers introduces a novel way of testing the predictive power of two loss reserving methodologies. Using a database created by the CAS that consists of hundreds of loss development triangles with outcomes, the volume begins by first testing the performance of the Mack model on incurred data and the Bootstrap Overdispersed Poisson model on paid data. As the emergence of Bayesian MCMC models has provided actuaries with an unprecedented flexibility in stochastic model development, the monograph then identifies some Bayesian MCMC (Markov Chain Monte Carlo) models that improve the performance over the above models. PLEASE NOTE that there is now a revised edition of this monograph (Monograph #8).
The monograph has been adopted on the syllabus for Exam 7.
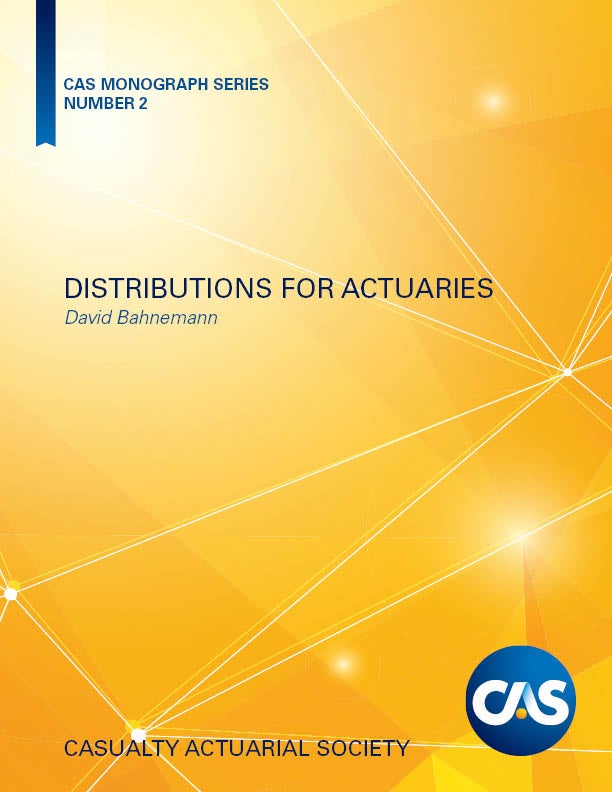
CAS Monograph No. 2 –Distributions for Actuaries
by David Bahnemann
In Distributions for Actuaries, David Bahnemann brings together two important elements of actuarial practice: an academic presentation of parametric distributions and the application of these distributions in the actuarial paradigm. The focus is on the use of parametric distributions fitted to empirical claim data to solve standard actuarial problems, such as creation of increased limit factors, pricing of deductibles, and evaluating the effect of aggregate limits.
The monograph has been adopted on the syllabus for Exam 8.
In Distributions for Actuaries, David Bahnemann brings together two important elements of actuarial practice: an academic presentation of parametric distributions and the application of these distributions in the actuarial paradigm. The focus is on the use of parametric distributions fitted to empirical claim data to solve standard actuarial problems, such as creation of increased limit factors, pricing of deductibles, and evaluating the effect of aggregate limits.
The monograph has been adopted on the syllabus for Exam 8.
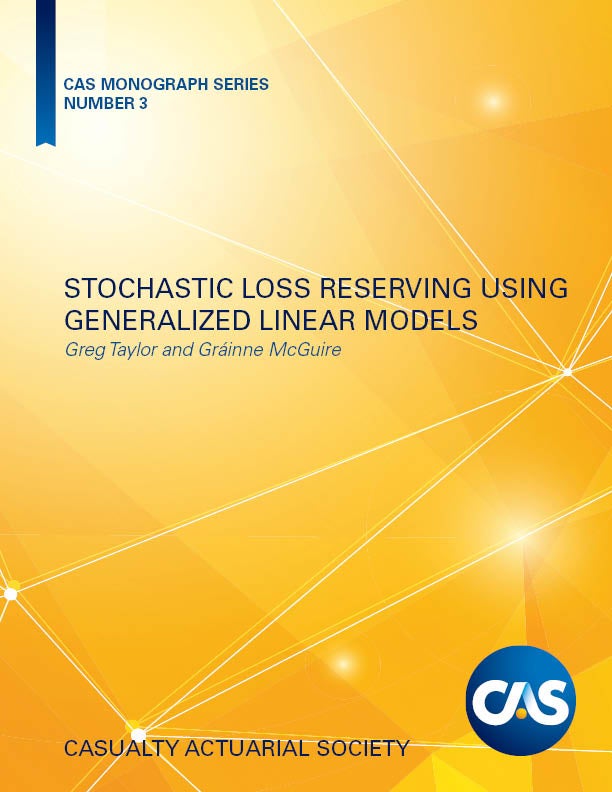
CAS Monograph No. 3 – Stochastic Loss Reserving Using Generalized Linear Models
by Greg Taylor and Gráinne McGuire
In this monograph, authors Greg Taylor and Gráinne McGuire discuss generalized linear models (GLM) for loss reserving, beginning with strong emphasis on the chain ladder. The chain ladder is formulated in a GLM context, as is the statistical distribution of the loss reserve. This structure is then used to test the need for departure from the chain ladder model and to consider natural extensions of the chain ladder model that lend themselves to the GLM framework.
The monograph has been adopted on the syllabus for Exam 7.
In this monograph, authors Greg Taylor and Gráinne McGuire discuss generalized linear models (GLM) for loss reserving, beginning with strong emphasis on the chain ladder. The chain ladder is formulated in a GLM context, as is the statistical distribution of the loss reserve. This structure is then used to test the need for departure from the chain ladder model and to consider natural extensions of the chain ladder model that lend themselves to the GLM framework.
The monograph has been adopted on the syllabus for Exam 7.
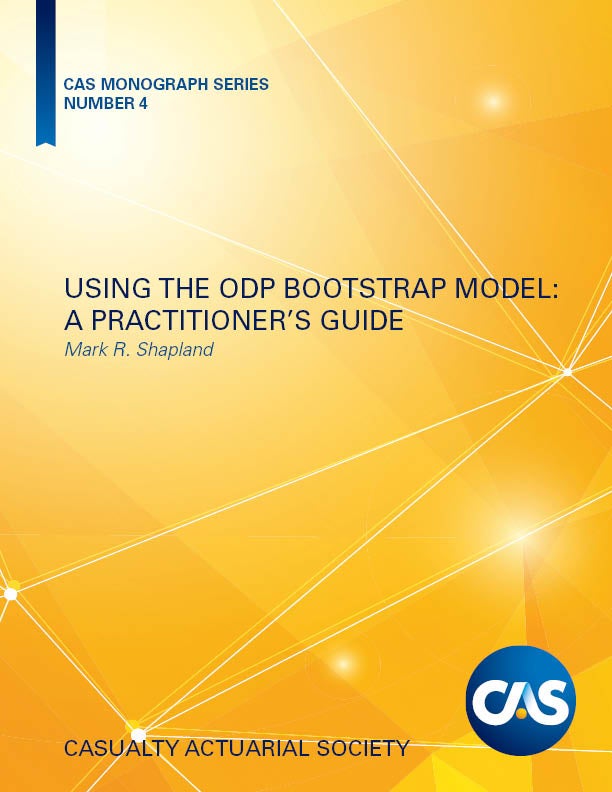
CAS Monograph No. 4 – Using the ODP Bootstrap Model: A Practitioner’s Guide
by Mark Shapland
In this monograph, Using the ODP Bootstrap Model: A Practitioner’s Guide, the fourth volume of the new CAS Monograph Series, author Mark R. Shapland, a Fellow of the CAS, discusses the practical issues and solutions for dealing with the limitations of ODP bootstrapping models, including practical considerations for selecting the best assumptions and the best model for individual situations. The focus is on the “practical” and the paper illustrates the diagnostic tools that an actuary needs to assess whether a model is working well.
The monograph has been adopted on the syllabus for Exam 7.
In this monograph, Using the ODP Bootstrap Model: A Practitioner’s Guide, the fourth volume of the new CAS Monograph Series, author Mark R. Shapland, a Fellow of the CAS, discusses the practical issues and solutions for dealing with the limitations of ODP bootstrapping models, including practical considerations for selecting the best assumptions and the best model for individual situations. The focus is on the “practical” and the paper illustrates the diagnostic tools that an actuary needs to assess whether a model is working well.
The monograph has been adopted on the syllabus for Exam 7.
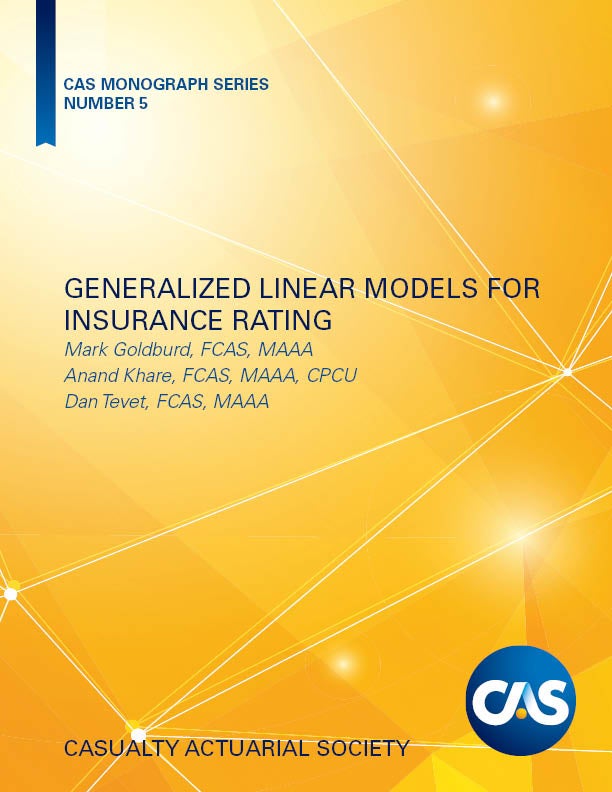
CAS Monograph No. 5 – Generalized Linear Models for Insurance Rating, 2nd edition
by Mark Goldburd, Anand Khare, Dan Tevet, and Dmitriy Guller
In this monograph, Generalized Linear Models for Insurance Rating, the fifth volume of the new CAS Monograph Series, authors Mark Goldburd, Anand Khare, Dan Tevet, and Dmitriy Guller have written a comprehensive guide to creating an insurance rating plan using generalized linear models (GLMs), with an emphasis on practical application. While many textbooks and papers exist to explain GLMs, this monograph serves as a “one-stop shop” for the actuary seeking to understand the GLM model-building process from start to finish. The monograph has been adopted on the syllabus for Exam 8.
In this monograph, Generalized Linear Models for Insurance Rating, the fifth volume of the new CAS Monograph Series, authors Mark Goldburd, Anand Khare, Dan Tevet, and Dmitriy Guller have written a comprehensive guide to creating an insurance rating plan using generalized linear models (GLMs), with an emphasis on practical application. While many textbooks and papers exist to explain GLMs, this monograph serves as a “one-stop shop” for the actuary seeking to understand the GLM model-building process from start to finish. The monograph has been adopted on the syllabus for Exam 8.
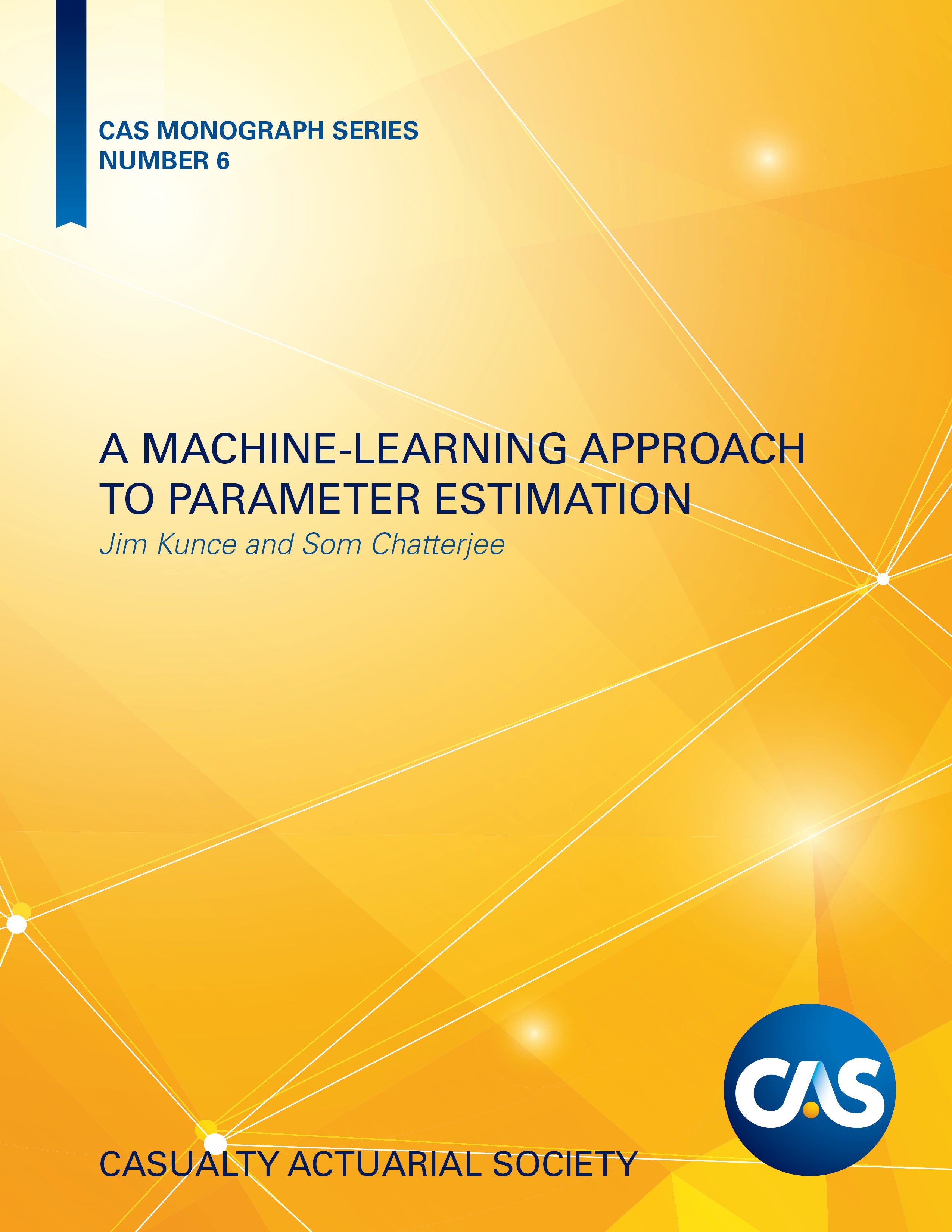
CAS Monograph No. 6 – A Machine-Learning Approach to Parameter Estimation
by Jim Kunce and Som Chatterjee
In this monograph, A Machine-Learning Approach to Parameter Estimation, the sixth volume of the CAS Monograph Series, CAS Fellows Jim Kunce and Som Chatterjee address the use of machine-learning techniques to solve insurance problems. Their model can use any regression-based machine-learning algorithm to analyze the nonlinear relationships between the parameters of statistical distributions and features that relate to a specific problem. Unlike traditional stratification and segmentation, the authors’ machine-learning approach to parameter estimation (MLAPE) learns the underlying parameter groups from the data and uses validation to ensure appropriate predictive power.
In this monograph, A Machine-Learning Approach to Parameter Estimation, the sixth volume of the CAS Monograph Series, CAS Fellows Jim Kunce and Som Chatterjee address the use of machine-learning techniques to solve insurance problems. Their model can use any regression-based machine-learning algorithm to analyze the nonlinear relationships between the parameters of statistical distributions and features that relate to a specific problem. Unlike traditional stratification and segmentation, the authors’ machine-learning approach to parameter estimation (MLAPE) learns the underlying parameter groups from the data and uses validation to ensure appropriate predictive power.
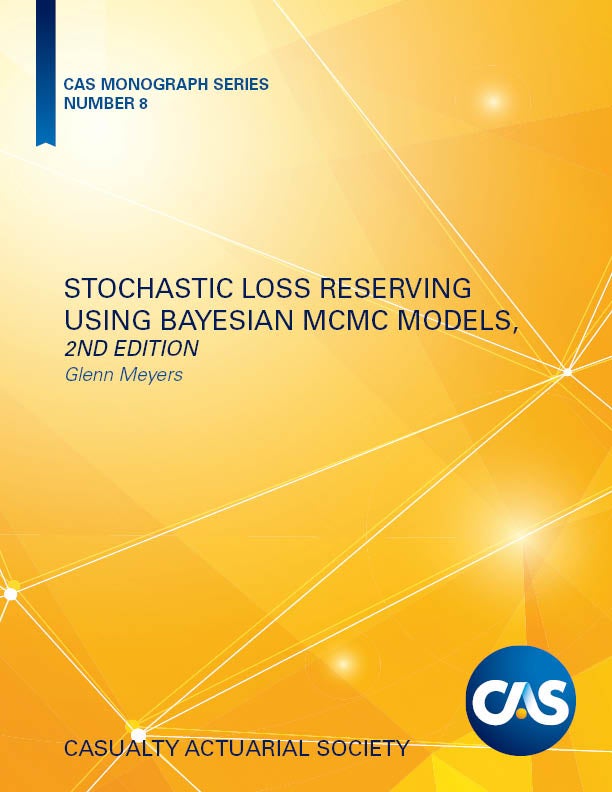
CAS Monograph No. 8 — Stochastic Loss Reserving Using Bayesian MCMC Models (2nd edition)
by Glenn Meyers
The emergence of Bayesian Markov Chain Monte-Carlo (MCMC) models has provided actuaries with an unprecedented flexibility in stochastic model development. Another recent development has been the posting of a database on the CAS website that consists of hundreds of loss development triangles with outcomes. This monograph begins by first testing the performance of the Mack model on incurred data, and the Bootstrap Overdispersed Poisson model on paid data. It then proposes Bayesian MCMC models that improve the performance over the above models. The features examined include (1) recognizing correlation between accident years in incurred data, (2) allowing for a change in the claim settlement rate in paid data, and (3) a unified model combining paid and incurred data. This monograph continues with an investigation of dependencies between lines of insurance and proposes a way to calculate a cost of capital risk margin.
The emergence of Bayesian Markov Chain Monte-Carlo (MCMC) models has provided actuaries with an unprecedented flexibility in stochastic model development. Another recent development has been the posting of a database on the CAS website that consists of hundreds of loss development triangles with outcomes. This monograph begins by first testing the performance of the Mack model on incurred data, and the Bootstrap Overdispersed Poisson model on paid data. It then proposes Bayesian MCMC models that improve the performance over the above models. The features examined include (1) recognizing correlation between accident years in incurred data, (2) allowing for a change in the claim settlement rate in paid data, and (3) a unified model combining paid and incurred data. This monograph continues with an investigation of dependencies between lines of insurance and proposes a way to calculate a cost of capital risk margin.
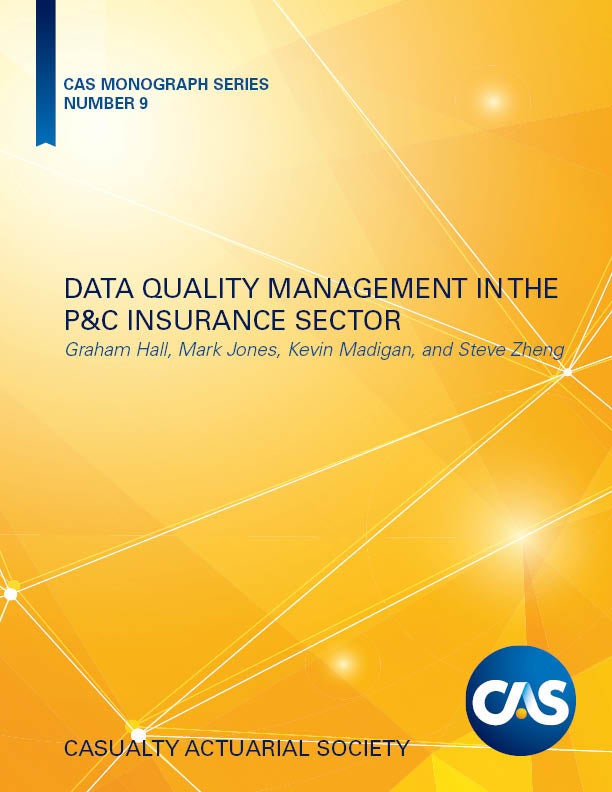
CAS Monograph No. 9 — Data Quality Management in the P&C Insurance Sector
by Graham Hall, Mark Jones, Kevin Madigan, and Steve Zheng
Reliable data has always been integral to P&C insurer operations, but the importance of data quality has increased significantly as new data sources and analytical methods, such as machine learning and artificial intelligence, have become available. The phrase “garbage in, garbage out” has never been more relevant, and actuaries increasingly must understand and quantify the impact that the quality of the data has on their work. This monograph begins in Section 1 with an introduction to the concept of data quality management, including a discussion of what is meant by data quality. Section 2 then discusses the impact of data quality on different actuarial processes and product lines, followed by a presentation of the current state of data quality within the P&C insurance market as informed by a survey of CAS members in Section 3. Section 4 then analyzes the treatment of data quality by the most significant global insurance regulatory regimes. In Section 5, the authors describe key considerations when designing a data quality management framework, including data architecture and technology/systems design; common data models, including the relational and NoSQL data models; and data governance. Building from the relational data model, the authors define a series of data anomaly types and use these to formally define data quality measures in Section 6. Finally, in Section 7, data quality improvement/imputation techniques are discussed and demonstrated on a sample insurance dataset. The sections are ordered with the reader’s intentions in mind. A focus on Sections 1 through 4 is recommended for the reader who is interested in an overview of data quality management and its importance. The reader who desires a more technical and practical overview of building a database and working with anomalous data should begin with Section 1 and then place more emphasis on Sections 5 through 7.
Reliable data has always been integral to P&C insurer operations, but the importance of data quality has increased significantly as new data sources and analytical methods, such as machine learning and artificial intelligence, have become available. The phrase “garbage in, garbage out” has never been more relevant, and actuaries increasingly must understand and quantify the impact that the quality of the data has on their work. This monograph begins in Section 1 with an introduction to the concept of data quality management, including a discussion of what is meant by data quality. Section 2 then discusses the impact of data quality on different actuarial processes and product lines, followed by a presentation of the current state of data quality within the P&C insurance market as informed by a survey of CAS members in Section 3. Section 4 then analyzes the treatment of data quality by the most significant global insurance regulatory regimes. In Section 5, the authors describe key considerations when designing a data quality management framework, including data architecture and technology/systems design; common data models, including the relational and NoSQL data models; and data governance. Building from the relational data model, the authors define a series of data anomaly types and use these to formally define data quality measures in Section 6. Finally, in Section 7, data quality improvement/imputation techniques are discussed and demonstrated on a sample insurance dataset. The sections are ordered with the reader’s intentions in mind. A focus on Sections 1 through 4 is recommended for the reader who is interested in an overview of data quality management and its importance. The reader who desires a more technical and practical overview of building a database and working with anomalous data should begin with Section 1 and then place more emphasis on Sections 5 through 7.
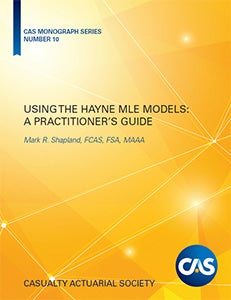
CAS Monograph No. 10 - Using the Hayne MLE Models: A Practitioner's Guide
by Mark Shapland
This monograph illustrates the practical implementation of the Hayne MLE modeling framework as a powerful tool for estimating a distribution of unpaid claims. It starts by reviewing the Hayne MLE modeling framework using a standard notation. Then it covers a number of practical data issues and addresses the diagnostic testing of the model assumptions. Next, it explores a variety of enhancements to the basic framework to allow the models to address other issues related to reserving and pricing risk. Finally, since no single model is perfect, ways to combine or credibility weight the Hayne MLE model results with various other models are explored in order to arrive at a “best estimate” of the distribution.
This monograph illustrates the practical implementation of the Hayne MLE modeling framework as a powerful tool for estimating a distribution of unpaid claims. It starts by reviewing the Hayne MLE modeling framework using a standard notation. Then it covers a number of practical data issues and addresses the diagnostic testing of the model assumptions. Next, it explores a variety of enhancements to the basic framework to allow the models to address other issues related to reserving and pricing risk. Finally, since no single model is perfect, ways to combine or credibility weight the Hayne MLE model results with various other models are explored in order to arrive at a “best estimate” of the distribution.
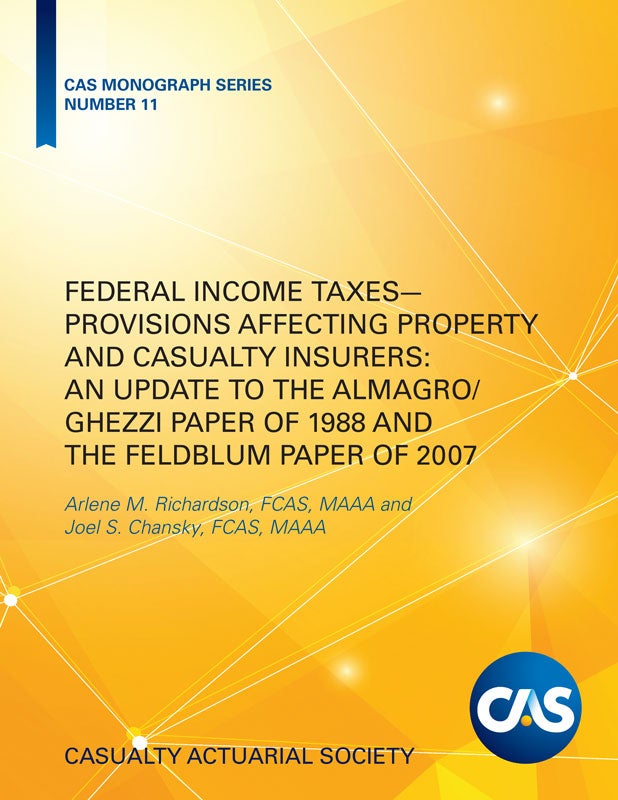
CAS Monograph No. 11- Federal Income Taxes — Provisions Affecting Property and Casualty Insurers: An Update to the Almagro/Ghezzi Paper of 1988 and the Feldblum Paper of 2007
by Arlene M. Richardson, FCAS, MAAA and Joel S. Chansky, FCAS, MAAA
This paper provides an overview of the key provisions in the 2017 Federal Income Tax Legislation (called the Tax Cuts and Jobs Act, and referred to as the “2017 FITL” in this paper) affecting property and casualty insurers, all of which are effective for tax years beginning after December 31, 2017. Some of the provisions affect all corporate taxpayers and some are unique to property and casualty insurers. The focus of the paper is on after-tax income and the strategies that property and casualty insurers may consider in optimizing after-tax income. The tax calculations in this paper are performed at a high level and are not intended to capture all of the nuances and details of an actual tax return. Rather, the examples and calculations are intended to illustrate the impact of the changes in the tax law, as well as to illustrate the impact of different investment and pricing strategies on after-tax income. The first section of the paper provides background information on the key federal tax changes of 1986 and 2017 and the impacts on property and casualty insurers. The Tax Reform Act of 1986 (referred to as the “1986 FITL” in this paper) was the last major change in the federal tax law prior to the 2017 FITL. Historical context is important in understanding where we have been. We also include a notional company’s income statement and balance sheet as of December 31, 2017, and use this as the basis for testing the impact of the various changes in the 2017 FITL. The second section of the paper focuses on the impact of the 2017 FITL on investment strategies of property and casualty insurers. The third section of the paper a) focuses on the changes made to the marginal tax rates and rules for discounting loss reserves of property and casualty insurers, and b) quantifies the impact of the changes on the notional company’s after-tax income. The fourth and final section of the paper discusses pricing considerations for property and casualty insurers and illustrates after-tax income (expressed as internal rates of return) both pre- and post-2017 FITL. A sample approach is provided for quantifying the percentage change in 2018 premium rates required to achieve the same internal rate of return as that based on pricing models that were used in 2017 prior to the passage of the 2017 FITL to promulgate 2018 premium rates, with only the change in the tax law as a “variable.”
This paper provides an overview of the key provisions in the 2017 Federal Income Tax Legislation (called the Tax Cuts and Jobs Act, and referred to as the “2017 FITL” in this paper) affecting property and casualty insurers, all of which are effective for tax years beginning after December 31, 2017. Some of the provisions affect all corporate taxpayers and some are unique to property and casualty insurers. The focus of the paper is on after-tax income and the strategies that property and casualty insurers may consider in optimizing after-tax income. The tax calculations in this paper are performed at a high level and are not intended to capture all of the nuances and details of an actual tax return. Rather, the examples and calculations are intended to illustrate the impact of the changes in the tax law, as well as to illustrate the impact of different investment and pricing strategies on after-tax income. The first section of the paper provides background information on the key federal tax changes of 1986 and 2017 and the impacts on property and casualty insurers. The Tax Reform Act of 1986 (referred to as the “1986 FITL” in this paper) was the last major change in the federal tax law prior to the 2017 FITL. Historical context is important in understanding where we have been. We also include a notional company’s income statement and balance sheet as of December 31, 2017, and use this as the basis for testing the impact of the various changes in the 2017 FITL. The second section of the paper focuses on the impact of the 2017 FITL on investment strategies of property and casualty insurers. The third section of the paper a) focuses on the changes made to the marginal tax rates and rules for discounting loss reserves of property and casualty insurers, and b) quantifies the impact of the changes on the notional company’s after-tax income. The fourth and final section of the paper discusses pricing considerations for property and casualty insurers and illustrates after-tax income (expressed as internal rates of return) both pre- and post-2017 FITL. A sample approach is provided for quantifying the percentage change in 2018 premium rates required to achieve the same internal rate of return as that based on pricing models that were used in 2017 prior to the passage of the 2017 FITL to promulgate 2018 premium rates, with only the change in the tax law as a “variable.”
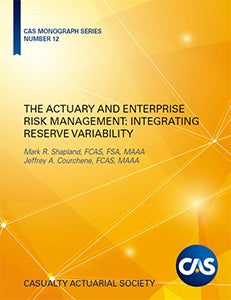
CAS Monograph No. 12 - The Actuary and Enterprise Risk Management: Integrating Reserve Variability
by Mark R. Shapland and Jeffrey A. Courchene
The development of a wide variety of reserve variability models has been primarily driven by the need to quantify reserve uncertainty. The Actuary and Enterprise Risk Management: Integrating Reserve Variability moves beyond quantification and explores other aspects of reserve variability that allow for a more complete integration of these key risk metrics into the larger enterprise risk management framework. It uses a case study to discuss and illustrate the process of integrating the output from periodic reserve and reserve variability analysis into the wider enterprise risk management processes. Consequences of this approach include the production of valuable performance indicators and a strengthening of the lines of communication between the actuarial function and other insurance functional departments, both of which are valuable to management.
The development of a wide variety of reserve variability models has been primarily driven by the need to quantify reserve uncertainty. The Actuary and Enterprise Risk Management: Integrating Reserve Variability moves beyond quantification and explores other aspects of reserve variability that allow for a more complete integration of these key risk metrics into the larger enterprise risk management framework. It uses a case study to discuss and illustrate the process of integrating the output from periodic reserve and reserve variability analysis into the wider enterprise risk management processes. Consequences of this approach include the production of valuable performance indicators and a strengthening of the lines of communication between the actuarial function and other insurance functional departments, both of which are valuable to management.